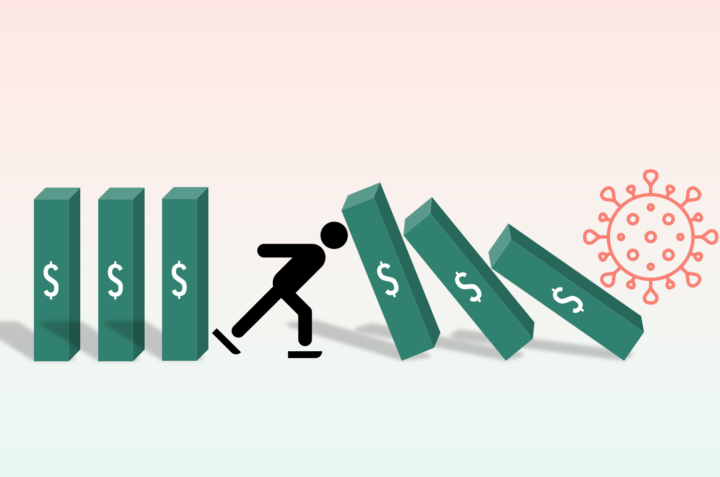
Shock Resilient Funding: A COVID Case Study9 min read
Don’t keep all your eggs in one basket is an English-language saying that conveys a simple piece of wisdom. One of the things that keeps a system strong and resilient is diversifying. Don’t make everything dependent on one thing. Whether that be not investing all your spare cash in Bitcoin, or an NGO having multiple funding streams, or a contestant on the One Million Pound Drop, you want to minimise your risk by spreading it out.
This is especially true when there is an external shock, and they don’t come any bigger or more comprehensive than the COVID-19 pandemic. This allows us to ask, and (somewhat) answer, an interesting question: does greater diversity in funding sources to a humanitarian sector make it more resilient to shocks?
The logic here is quite simple.
- External shock hits, causing donors to reallocate funding
- Risk of big funding drop if sector is overdependent on one donor
- One donor decides to withdraw or reduce funding
- Big funding drop
And vice versa. Funding from multiple sources minimises the impact of the shock by spreading the risk out across various donors.
What we’ve found so far is that if a sector is more dependent on just a few donors, then it is less resilient. What this meant for COVID-19 was that Logistics and Food Security weren’t well prepared (and suffered declines in funding), whereas sectors which had greater diversity in funding, like Education and Protection, weathered the storm better.
Humanitarian Regression
Did funding for some sectors regress more than others due to lack of diversity in funding sources? To find out, we’re going to use multiple linear regression. For non-stats folk, this is a method of examining the effect of multiple variables on another variable.
We have 13 sectors that we are examining. We’re choosing to exclude Agriculture, Early Recovery, Emergency Telecommunications and Mine Action as these are special cases. They are low value sectors and their volatility creates large swings in percentage growth, which creates noise in the analysis.
Ideally we would have a larger sample size than we have. But thankfully there has only been one global pandemic in recent years. So we’re stuck with only 13 sectors. What this means is that we should be quite cautious about overinterpreting these results and placing huge amounts of confidence in the results. But it might still give us an insight.
Going into our regression model is the variable for which we want to see if there is any change: percentage growth in funding to a sector in 2020 (vs. 2019). We also have four independent variables that could affect sector growth:
- Number of donors – this tests whether just the number of donors has an impact
- Herfindahl Hirschman Index (HHI) score – this is a measure of how concentrated the sector is, or how dependent the sector is on one or a few donors. If 100% of funding came from one donor, this would equal 10,000. If 50% of funding came from two donors each, this would equal 5,000. See here for the calculation method.
- Previous growth rate of the sector – this seems like one of the most obvious things to test. The previous growth rate of the sector could have an affect on the growth rate in a crisis. We’ll test the compound annual growth rate between 2016 and 2019.
- Funding required (funding requirement) change compared to the previous year – this tests whether an increase in overall funding asked for makes a difference. This is like a proxy for the importance of that sector in the pandemic. For example, we’d expect the ‘Health’ requirement to have increased in a health crisis such as COVID-19.
Of these four variables, we’re most interested in the first two for our question. But by including the other two, we’re accounting for their effect in the model. If we didn’t do this then the results for the number of donors or HHI score would be different and would not take into account the effects of the other two variables. We’ve run all possible combinations of these variables and will show the best fitting model below (best subsets regression).
We’re only including funding that is going to a response plan, as we can be sure that this is going to an identified humanitarian need, and we’re excluding pledges.
The impact of funding diversity
All other things being equal, and with these results caveated with the above (low sample size):
- Reduced dependence on a few donors equals more funding. In other words, spreading the market share across more donors increases funding in the face of a shock. For every 1000 point decrease (that’s reduced dependence) in the HHI score, a sector’s growth during the shock was 11.4% better than it would have otherwise been.
- More funding required equals more funding. For every 1% increase in the funding required, you would expect to see 0.45% increase in funding during the external shock.
- More medium-term growth equals more funding. For every additional 1% of medium-term growth, you would expect to see 0.3% increase in funding during the external shock.
One result that is much less certain is that more donors equals more funding. Not only do we only have 13 sectors in our analysis, but the p-value of the ‘number of donors’ variable is 0.2, which is quite high.
A p-value is the probability of any effects being random chance. Thresholds of 0.1 (and under), 0.05 (and under), and 0.01 (and under), are usually used to determine ‘statistical significance’. So 0.2 is quite high, particularly from the small sample size. The coefficient is the average change in the dependent variable, based on one unit of change in the independent variable. We’ve summarised these in an easy-to-understand form on the above bullet point list.
Coefficient | P-Value | |
Intercept | -0.2647 | 0.09 |
Requirement growth | 0.4549 | 0.02 |
2016-2019 Year on Year Growth | 0.3041 | 0.01 |
HHI Score | -0.0001 | 0.07 |
Number of donors | 0.0028 | 0.20 |
We won’t come to any conclusions on this here, and we need to think more about how we can test this. If we ignored these caveats, however (which is a big leap), and assumed that the number of donors was significant, our model tells us that for every additional donor in a sector, growth during the external shock would be 0.28% higher. However, it’s a big leap to ignore those caveats just because of the strong logical argument of more donors equalling a resilient funding system.
This model is by no means complete. The adjusted R2 – that’s the amount of variance explained by these four variables – is 0.79, meaning we still can’t account for over 20% of the effects on growth in the year of an external shock. In reality, the adjusted R2 is probably lower given the low sample size, so we can’t be too sure about the effects of the four variables.
But the model does an okay job. It gets right whether a sector grows or shrinks 11 out of 13 times (though doesn’t necessarily get the magnitude correct). The full results of this are in the table below so you can check it out by sector.
We’ve also ordered the table by HHI, sorting from top HHI scores (dependence on small number of donors), to low HHI scores (diverse sector). It’s noticeable that the five sectors with the highest HHI all experienced negative growth in 2020.
It also tells us that certain sectors overperformed compared to what they would be expected to receive based on our (flawed) model. Logistics only experienced -14% growth, versus -29% that would be expected. Similarly Health experienced 42% growth, when they’d only be expected to increase by 31% based on the model.
But other sectors underperformed. WASH is a sector with a large increase in funding required, large number of donors, solid previous medium-term growth, and diversity/low dependence on small number of donors. They would have been expected to grow by 7%, but they actually shrunk by 13%. Similarly, Nutrition was expected to shrink by 17% but actually shrunk by 33%.
What this all means
In no way are we saying these effects are precise (e.g. growth increasing by x% if this or that variable increases). Don’t focus on that. The model is flawed in many ways. Our sample size is way too small, and we’re definitely missing some other variables that will have an effect.
There’s a well known quote, that “All models are wrong, but some are useful”. Our model is wrong, but hopefully useful.
What we should focus more on is the basic insight into the direction of the effect. Less dependence on a small number of donors equals less impact of the shock. Higher medium-term growth equals less impact of the shock. Higher funding requirement equals less impact of the shock (although really that’s about the relative importance of a sector to the shock more than anything else, and not something we can necessarily affect too much). And maybe, more donors equals less impact of the shock – more investigation is needed on that one.
Taking this insight into action
Sectors should be looking at their HHI score, and think about diversifying their dependence on certain donors. This includes Food Security (HHI score of 3,108), Logistics (1,989), Multi-Sector (1,980), and Nutrition (1,891). For example, the US Government contributes 54% of Food Security funding. Reducing this percentage (which means increasing the percentage of funding received from other donors) will hopefully increase funding, and also increase resilience when it comes to external shocks.
Sectors could also look expanding the numbers of donors. Notably CCCM (25 donors), Logistics (25 donors), GBV (26 donors), and Child Protection (31 donors). For comparison, the median number across the sectors examined here is 59 donors. We didn’t come to any conclusions through the analysis on this, but common sense says suggests this wouldn’t be a bad thing to do.
So while acknowledging the flaws in the model, we hope it’s useful in providing some highly-caveated evidence of the basic insight of “don’t put all your eggs in one basket”. The results here provide a slight hint and a careful nod towards a strategy that some sectors might want to consider to make sure the next big shock doesn’t strike so hard.
Sources and Annex
Numbers on this story may differ from numbers on the ‘Sector’ pages. This is not an error, but simply due to different data extraction dates from FTS.
FTS. Data Search. All 2016 funding. Retrieved from here
FTS. Data Search. All 2017 funding. Retrieved from here
FTS. Data Search. All 2018 funding. Retrieved from here
FTS. Data Search. All 2019 funding. Retrieved from here
FTS. Data Search. All 2020 funding. Retrieved from here
FTS. 2016 Appeals. https://fts.unocha.org/appeals/overview/2016
FTS. 2017 Appeals. https://fts.unocha.org/appeals/overview/2017
FTS. 2018 Appeals. https://fts.unocha.org/appeals/overview/2018
FTS. 2019 Appeals. https://fts.unocha.org/appeals/overview/2019
FTS. 2020 Appeals. https://fts.unocha.org/appeals/overview/2020